BioLogistics and the Struggle for Efficiency
This webpage provides supplementary information to the Manuscript
external page BioLogistics and the Struggle for Efficiency: Concepts and Perspectives
by Prof. Dr. Dirk Helbing, Prof. Dr. Andreas Deutsch, Dr. Stefan Diez, Dr. Karsten Peters, Dr. Yannis Kalaidzidis, Dr. Kathrin Padberg, Dr. Stefan Lämmer, Anders Fredrik Johansson, Prof. Dr. Georg Breier, Dr. Frank Schulze, Prof. Dr. Marino Zerial
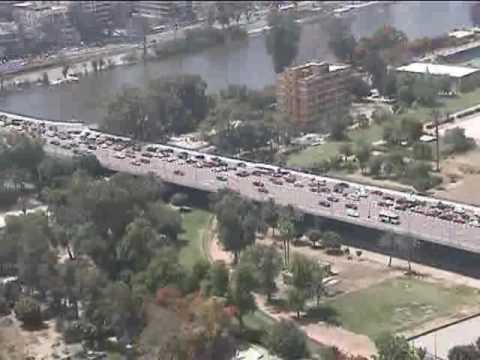
Video SV 1 - Breakdown of free traffic flow:
Video recording of the spontaneous breakdown of free traffic flow at a factually isolated on-ramp, merging with an intra-urban freeway next to the public observation tower in Cairo, Egypt.
During the recording, there were free flow conditions downstream of the on-ramp. The small inflow via the ramp reproducibly triggered the breakdown of traffic flow and the emergence of upstream moving stop-and-go waves. Qualitatively very similar traffic patterns are generated by the microscopic traffic simulator available at: external page http://www.traffic-simulation.de.
For the breakdown of free traffic flow in a closed, circular system see the experiment by Y. Sugiyama et al. displayed at: external page http://www.iop.org/EJ/mmedia/1367-2630/10/3/033001/.
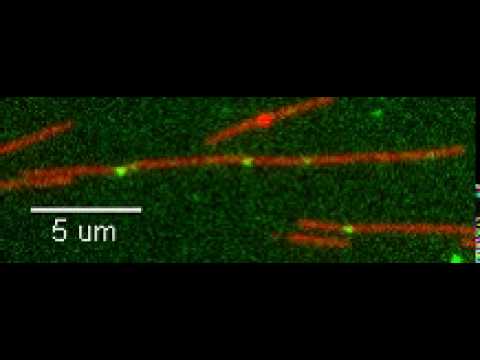
Video SV 2 - Movement of motor proteins along microtubules:
Experimental results showing single kinesin-1 molecules (fluorescently labelled with the green-fluorescent protein, green) moving along surface-immobilized microtubules (fluorescently labelled with rhodamine, red). Microtubules are hollow protein tubes (with a diameter of about 25 nanometer) originating from the circular self-assembly of about 13 long chains of dimeric tubulin proteins, the so-called protofilaments. Due to an inherent asymmetry in the tubulin dimers, microtubules possess a structural polarity, which is recognized by motor proteins specifically stepping towards the one or the other direction. In the experiment shown by this video, microtubules have been reconstituted in vitro by self-assembly from dimeric tubulin proteins. The filaments were then bound to a glass-coverslip by rhodamine-antibodies and total-internal reflection fluorescence microscopy was used to image the movement of individual kinesin-1 motors along the microtubules in the presence of ATP. Images were acquired with a frequency of 10 frames per second and the playback speed corresponds to real time (scale bar denotes a length of 5 µm).
In this movie, the kinesin-1 motors are seen to follow a certain direction (towards the microtubule plus ends) on each microtubule. The typical velocity of this motion is about 800 nm/s. While kinesin-1 is a processive motor (i.e. the motor is capable of performing multiple consecutive steps after encountering the microtubule), its run length is limited. After about 100 steps, each of 8-nm length, the motors dissociate from the microtubule, diffuse in solution and are available for binding again at another position. If the surface of the microtubule is blocked by other proteins, the motors will stall and finally detach at the location of the obstacle. In case of transport by single motors, this causes a highly interruptive transport process. If, on the other hand, multiple motors are engaged, the obstacles can lead to a significant slow-down of the transport velocity.
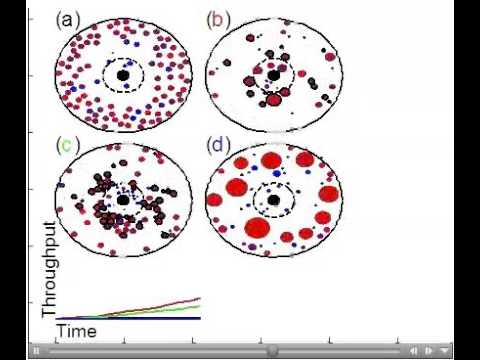
Video SV 3 - Endosome traffic:
Animation of simplified endosome traffic, illustrating some of the experimentally observed features. Endosomes (small coloured circles) are generated at the cell surface at a certain rate by internalizing "cargo". They transport materials to and from the so-called peri-nuclear region (area inside the dashed circle), which is located around the cell nucleus (black circle). In our simulation, endosomes are assumed to contain two kinds of materials, A (red) and B (blue), which coexist for an extended time period. Their relative concentration determines the colour shade and is random in the beginning. Endosomes with a high concentration of material B may fuse with the plasma membrane of the cell surface, thereby releasing their cargo to the outside of the cell. Material A, in contrast, is consumed ("degraded") in the peri-nuclear region at a rate proportional to the surface of the endosome.
We compare four scenarios: (a) Endosomes move in a diffusive manner only. As reflected by the high crowdedness in the cell and the low throughput (black time-dependent line at the bottom of the video), the transport of material A towards the nucleus is quite inefficient. (b) Low crowdedness and high throughput (red time-dependent line) are reached by several "tricks" that the cell uses to improve endosome traffic: 1. Endosomes are assumed to fuse when they happen to touch each other, which generates a bigger endosome containing the materials A and B of the previous two endosomes. 2. Material B is continuously extracted by asymmetric fission, where material B is removed, while material A is retained. By the alternation of fusion and fission, endosomes perform progressive sorting. 3. The cell contains radial tracks (microtubules), which are used for directed transport. Directed transport (visualized by black circles around the coloured endosomes) starts, when the endosomes happen to touch a respective microtubule by diffusion, and it ends, as endosomes are "kicked off" from the microtubules by various intracellular processes at a certain rate q. Between the phases of directed transport, endosomes move in a diffusive manner, otherwise endosomes with a concentration of material B higher than some threshold value y (here: y=50%) are assumed to be transported towards the cell surface (by molecular motors); endosomes with a concentration of material A higher than 1-y are assumed to be moved towards the peri-nuclear region. (c) Same as scenario (b), including phases of directed motion, but without fusion and fission processes (see green time-dependent line for the resulting flux of material A towards the peri-nuclear area). One observes mutual obstructions of endosomes and a high crowdedness in the cell. (d) Same as scenario (b), including fusing and fission, but without the mechanism of directed motion (see blue time-dependent line). Endosomes obviously grow too big and do not reach an efficient transport of material A. One clearly needs a combination of all three mechanisms ("tricks") mentioned above.
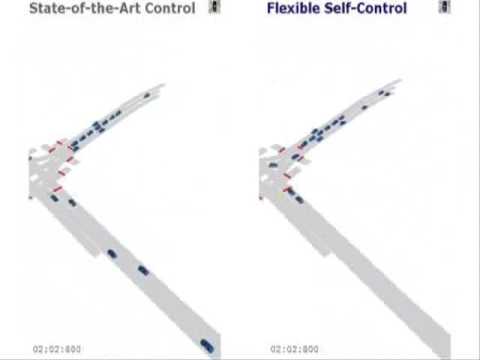
Video SV 4 - Traffic light control of vehicle flows in urban networks:
Microscopic simulation of traffic in the city centre of Dresden, Germany, using the simulation software VISSIM of PTV and empirically substantiated traffic data. Left: The current, state-of-the-art traffic control periodically provides green waves to vehicles in both major flow directions along the arterials. Before vehicles enter the coordinated arterial, they are queued at the first traffic light to form large platoons for the subsequent service by the green wave. When buses or trams need to cross the arterial, they are stopped until the vehicle platoons have passed. Right: The flexible self-control principle tries to serve each mode of traffic as soon as possible. This leads to shorter vehicle platoons and facilitates a more flexible service, utilizing gaps in the flow. Thereby, the self-organized traffic control manages to significantly reduce delays to all modes of transport. This is reflected by a smaller resulting vehicle density and by an earlier passage of intersections by arriving trams.
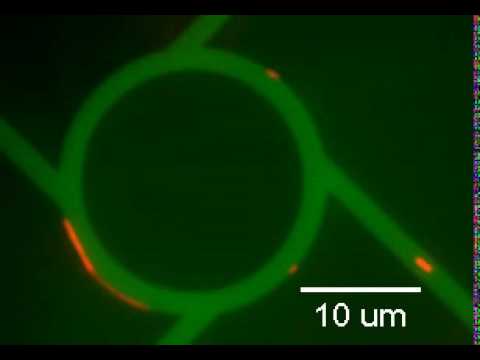
Video SV 5 - Towards logistics on the nano-scale:
Time-lapse series of fluorescence images showing the kinesin-driven, unidirectional movement of a rhodamine-labeled microtubule (red) along a chemically and topographically structured Si-chip. The bottom of the channels (green), whose depths are 300 nm, is coated with kinesin motor proteins. The surrounding regions are blocked by polyethylene glycol.
This movie has been generated in collaboration with R. M. M. Smeets, M. G. L. van den Heuvel, and C. Dekker (Delft University of Technology, The Netherlands). For further details, see also: Diez, S., Helenius, J. H. & Howard, J. Biomolecular motors operating in engineered environments, in Nanobiotechnology: Concepts, Applications and Perspectives (Wiley, Weinheim, 2004), Chap. 13, pp. 185-199, and van den Heuvel, M. G. L., Butcher, C. T., Smeets, R. M. M., Diez, S. & Dekker, C. High rectifying efficiencies of microtubule motility on kinesin-coated gold nanostructures, Nano Lett. 5(6), 1117-1122 (2005).
Table 1: Compilation of information on various logistic networks in nature and technology. We propose a comparative approach to these systems, which are traditionally addressed by separate disciplines of science. Both, in biological and technological networks, one can distinguish transport infrastructures ("tracks") and transported materials ("cargo"). Transport either happens in a continuous way (as for conveyor belts or blood flows) or by discrete, mobile units (like trucks or motor proteins). If the infrastructure consists of solid physical tracks, there are even similarities in terms of system sizes and topology. We believe that this fact is closely related to the limited possibilities to connect tracks to nodes in 2-dimensional or 3-dimensional space, respectively, i.e. the number of outgoing and incoming connections is quite limited.
Whereas the first columns of the table provide an overview of the type of network by giving information on the general purpose of the corresponding transport system, the used transport mechanism, the transported material ("cargo"), and the transport machinery, the remaining columns summarize some facts about the observed network topology and performance. We give an estimate of the network size (area), the typical number of nodes and links in the network, and finally the maximum node degree (indicating the maximum number of links meeting in a node) and clustering coefficient (the average probability that two neighbours of a given node are also neighbours of each other). Finally, some information on the transport performance of the respective networks is listed.
The blank fields in this table indicate that a logistics approach is not common in all concerned scientific disciplines.
Sources:
- Bebber, D. P., Darrah, P. R., Hynes, J., Boddy, L. & Fricker, M. D. Biological solutions to transport network design. Proc. R. Soc. B 274, 2307–2315 (2007).
- West, G. B., Brown, J. H. & Enquist, B. J. The fourth dimension of life: Fractal geometry and allometric scaling of organisms. Science 284, 1677–1679 (1999).
- Snodderly, D. M., Weinhaus, R. S. & Choi, J. C. Neural-vascular relationships in central retina of Macaque monkeys (Macaca fascicularis). J. Neurosci. 12(4), 1169–1193 (1992).
- Alberts, B. et al. Molecular Biology of the Cell (Garland Science, London, 2002).
- Howard, J. Mechanics of Motor Proteins and the Cytoskeleton (Sinauer Associates, Sunderland, 2001).
- Lämmer, S., Gehlsen, B. & Helbing, D. Scaling laws in the spatial structure of urban road networks. Physica A 363, 89–95 (2006).
- Analysis of different airport baggage handling systems, based on data that were kindly provided by Siemens.
- Analysis of 10 different inhouse material handling networks, based on data that were kindly provided by SCA Packaging.
- See e.g. Brandimarte, P. & Zotteri, G. Introduction to Distribution Logistics (Wiley, New York, 2007).
Detailed Author Information
(Note: The information might have changed. Please double-check with the websites of institutes and given authors.)
Prof. Dr. Dirk Helbing
ETH Zurich, UNO D11
Universitätstrasse 41
8092 Zurich, Switzerland
http://www.soms.ethz.ch
Phone +41 44 632 88 81
Fax +41 44 632 17 67
and
Collegium Budapest – Institute for Advanced Study
Szentharomsag utca 2
1014 Budapest, Hungary
Dr. Stefan Diez
Max Planck Institute of Molecular Cell Biology and Genetics
Pfotenhauerstrasse 108
01307 Dresden, Germany
http://www.mpi-cbg.de/~diez
Phone +49 351 210 2521
Fax +49 351 210 2020
Dr. Yannis Kalaidzidis
Max Planck Institute of Molecular Cell Biology and Genetics
Pfotenhauerstr. 108
01307 Dresden, Germany
http://www.mpi-cbg.de
Phone +49 351 210 2653
Fax +49 351 210 1389
and
A. N. Belozersky Institute of Physico-Chemical Biology
Moscow State University
Moscow 119899, Russia
Dr. Stefan Lämmer
Faculty of Transportation and Traffic Sciences "Friedrich List"
Technische Universität Dresden
Andreas-Schubert-Str. 23
01062 Dresden, Germany
http://traffic.stefanlaemmer.de
Phone +49 179 328 1231
Fax +49 351 463 36809
Prof. Dr. Georg Breier
Faculty of Medicine Carl Gustav Carus
Technische Universität Dresden
Fetscherstr. 74
01307 Dresden, Germany
http://www.tu-dresden.de/medecb/
Phone +49 351 458 5278
Fax +49 351 458 5814
Prof. Dr. Andreas Deutsch
Dept. for Innovative Methods of Computing (IMC)
Center for Information Services and High Performance Computing
Technische Universität Dresden
01062 Dresden, Germany
external page http://theobio.mtbio.de/imc/
Phone +49 351 463 31943
Fax +49 351 463 38245
Dr. Karsten Peters
Institute for Transport and Economics & Center for Information Services and High Performance Computing
Technische Universität Dresden
Andreas-Schubert-Str. 23
01062 Dresden, Germany
external page http://tu-dresden.de/Members/karsten.peters
Phone +49 351 463 31944
Fax +49 351 463 36809
Dr. Kathrin Padberg
Institute for Transport and Economics & Center for Information Services and High Performance Computing
Technische Universität Dresden
Andreas-Schubert-Str. 23
01062 Dresden, Germany
external page http://tu-dresden.de/Members/kathrin.padberg/
Phone +49 351 463 38776
Fax +49 351 463 36809
Anders Fredrik Johansson
ETH Zürich, UNO D11
Universitätstrasse 41
8092 Zurich, Switzerland
http://www.soms.ethz.ch
Phone +41 44 632 0239
Fax +41 44 632 1294
Dr. Frank Schulze
Technische Universität Dresden
Professur für Technische Logistik
01062 Dresden, Germany
external page http://tu-dresden.de/mw/tla
Phone +49 351 463 32537
Fax +49 351 463 35499
Prof. Dr. Marino Zerial
Max Planck Institute of Molecular Cell Biology and Genetics
Pfotenhauerstrasse 108
01307 Dresden, Germany
external page http://www.mpi-cbg.de
Phone +49 351 210 2636
Fax +49 351 210 1389
Suggested Reading
Bio-Inspired Approaches
1. Nachtigall, W. Bionik (Springer, Berlin, 2002).
2. Aldersey-Williams, H. Towards biomimetic architecture. Nature Mater. 3, 277–279 (2004).
3. Barthlott, W. & and C. Neinhuis, C. Purity of the sacred lotus, or escape from contamination in biological surfaces. Planta 202, 1–8 (1997).
4. Wainwright, S. A., Vosburgh, F. & Hebrank, J. H. Shark Skin: Function in Locomotion. Science 202, 747–749 (1978).
5. Autumn, K. et al. Adhesive force of a single gecko foot-hair. Nature 405, 681–685 (2000).
6. Autumn, K. et al. Evidence for van der Waals adhesion in gecko setae. Proc. Natl. Acad. Sci. USA 99, 12252–12256 (2002).
7. Dragnea, B. Unnatural life. Nature Mater. 7, 102–104 (2008).
8. Lee, S. & Spencer, N. D. Sweet, hairy, soft, and slippery. Science 319, 575–576 (2008).
9. Murphy, W. L. & Mooney, D. J. Molecular-scale biomimicry. Nature Biotechnol. 20, 30–31 (2002).
10. Saraniti, M. Designing biomimetic nanomachines. Nature Nanotechnol. 3, 647–648 (2008).
11. Camazine, S. et al. Self-Organization in Biological Systems (Princeton University, Princeton, 2001).
12. Vicsek, T. Fluctuations and Scaling in Biology (Oxford University Press, New York, 2001).
13. Keener, J. & Sneyd, J. Mathematical Physiology (Springer, New York, 2004).
14. Segel, L. A. & Cohen, I. R. (eds) Design Principles for the Immune System and Other Distributed Autonomous Systems (Oxford University Press, New York, 2001).
Evolutionary Approaches
15. Bentley, P. J. (ed) Evolutionary Design by Computers (Morgan Kaufmann, San Francisco, 1999).
16. Banzhaf, W. et al. From artificial evolution to computational evolution: a research agenda. Nature Rev. Genet. 7, 729–735 (2006).
17. Basanta, D. Using Genetic Algorithms to Evolve Microstructures (PhD thesis, University of London, 2005).
18. Kauffman, S. A. The Origins of Order: Self-Organization and Selection in Evolution (Oxford University Press, Oxford, 1993).
Swarm Intelligence
19. Bonabeau, E., Dorigo, M. & Theraulaz, G. Swarm Intelligence. From Natural to Artificial Systems (Oxford University Press, Oxford, 1999).
20. Blum, C. & Merkle, D. (eds) Swarm Intelligence. Introduction and Applications (Springer, Berlin, 2008).
21. Dorigo, M. & Stuetzle, T. Ant Colony Optimization (MIT Press, Cambridge, 2004).
22. Kennedy, J. & Eberhart, R. C. Swarm Intelligence (Morgan Kaufmann, San Francisco, 2001).
23. Engelbrecht, P. Fundamentals of Computational Swarm Intelligence (Wiley, London, 2007)
24. Nolfi, S. & Floreano, D. Evolutionary Robotics (MIT Press, Cambridge, 2000).
Self-organization, Synchronization, Coordination, and Cooperation
25. Nicolis, G. & Prigogine, I. Self-Organization in Nonequilibrium Systems: From Dissipative Structures to Order through Fluctuations (Wiley, New York, 1977).
26. Haken, H. Synergetics. An Introduction (Springer, Berlin, 1978).
27. Mikhailov, A. S. Foundations of Synergetics I: Distributed Active Systems (Springer, Berlin, 1990).
28. Di Marzo Serugendo, G., Karageorgos, A., Rana, O. F. & Zambonelli, F. (eds) Engineering Self-Organising Systems (Springer, Berlin, 2004).
29. Caponetto, R. Advanced Topics on Cellular Self-Organizing Nets and Chaotic Nonlinear Dynamics to Model and Control Complex Systems (World Scientific, Singapore, 2008).
30. Helbing, D., Buzna, L., Johansson, A. &Werner, T. Self-organized pedestrian crowd dynamics: Experiments, simulations, and design solutions. Transportation Sci. 39(1), 1–24 (2005).
31. Helbing, D., Siegmeier, J. & Lämmer, S. Self-organized network flows. Netw. Heterog. Media 2(2), 193–210 (2007).
32. Pikovsky, A., Rosenblum, M. & Kurths, J. Synchronization: A Universal Concept in Nonlinear Sciences (Cambridge University Press, Cambridge, 2008).
33. Arenas, A., Díaz-Guilera, A., Kurths, J., Moreno, Y. & Zhou, C. Synchronization in complex networks. Phys. Rep. 469, 93–153 (2008).
34. Glass, L. Synchronization and rhythmic processes in physiology. Nature 410, 277-284 (2001).
35. Axelrod, R. The Evolution of Cooperation (Basic, New York, 1984).
36. Nowak, M. A. Five rules for the evolution of cooperation. Science 314: 1560–1563 (2006).
37. Marcozzi, A. & Hales, D. Emergent social rationality in a peer-to-peer system. Adv. in Complex Systems 11(4), 581–595 (2008).
38. Helbing, D. A mathematical model for behavioral changes by pair interactions. In Economic Evolution and Demographic Change. Formal Models in Social Sciences (eds Haag, G., Mueller, U. & Troitzsch, K. G.) 330–348 (Springer, Berlin, 1992).
39. Helbing, D. & Yu, W. The outbreak of cooperation among success-driven individuals under noisy conditions. Proc. Natl. Acad. Sci. USA, in print (2009).
40. Kempf, K. G. Complexity and the enterprise: The illusion of control. In Managing Complexity (ed Helbing, D.) 57–87 (Springer, Berlin, 2008).
Related Approaches in Logistics, Production and Urban Traffic Control
41. Armbruster, D., Kaneko, K. & Mikhailov, A. S. (eds) Networks of interacting machines. Production Organization in Complex Industrial Systems and Biological Cells (World Scientific, Singapore, 2005).
42. Wang, W., Rivera, D. E. & Kempf, K. G. Model predictive control strategies for supply chain management in semiconductor manufacturing. Int. J. Production Economics 107, 56–77 (2007).
43. Radons, G. & Neugebauer, R. (eds) Nonlinear Dynamics of Production Systems (Wiley VCH, Weinheim, 2004).
44. Armbruster, D., de Beer, C., Freitag, M., Jagalski, T. & Ringhofer, C. Autonomous control of production networks using a pheromone approach. Physica A 363, 104-114 (2006).
45. Kumar, R., Tiwari, M. K. & Shankar, R. Scheduling of flexible manufacturing systems: an ant colony optimization approach. Proc. Instn. Mech. Engrs. B: J. Engineering Manufacture 217, 1443-1453 (2003).
46. Valckenaers, P., Van Brussel, H., Kollingbaum, M. & Bochmann, O. Multi-agent coordination and control using stigmergy applied to manufacturing control. In Multi-Agent Systems and Applications, LNAI 2086 (eds Luck, M. et al.) 317–334 (Springer, Berlin, 2001).
47. Duffie, N. A., Roy, D. & Shi, L. Dynamic modelling of production networks of autonomous work systems with local capacity control. CIRP Annals – Manufacturing Technology 57, 463-466 (2008).
48. Prabhu, V. V., Duffie, N. A. & Wysk, R. A. Special issue: Distributed control of manufacturing systems. Journal of Manufacturing Systems 26(2), 63ff (2007).
49. Ueda, K., Hatono, I., Fujii, N. & Vaario, J. Reinforcement learning approaches to biological manufacturing systems. Annals of the CIRP 49(1), 343–346 (2000).
50. Lämmer, S., Kori, H., Peters, K. & Helbing, D. Decentralised control of material or traffic flows in networks using phase-synchronisation. Physica A 363, 39–47 (2006).
51. Helbing, D., Seidel, T., Lämmer, S. & Peters, K. Self-organization principles in supply networks and production systems. In Econophysics and Sociophysics - Trends and Perspectives Pages (eds Chakrabarti, B. K., Chakraborti, A. & Chatterjee, A.) 535–558 (Wiley, Weinheim, 2006).
52. Seidel, T., Hartwig, J., Sanders, R. L. & Helbing, D. An agent-based approach to self-organized production. In Swarm Intelligence. Introduction and Applications (eds Blum, C. & Merkle, D.) 219–252 (Springer, Berlin, 2008).
53. Papageorgiou, M., Diakaki, C., Dinopoulou, V., Kotsialos, A. & Wang Y. Review of road traffic control strategies. Proc. IEEE 91(12), 2043-2067 (2003).
54. Kosmatopoulos, E. et al. International comparative field evaluation of a traffic-responsive signal control strategy in three cities. Transpn. Res. A 40, 399-413 (2006).
55. Helbing, D. & Lämmer, S. Verfahren zur Koordination konkurrierender Prozesse oder zur Steuerung des Transports von mobilen Einheiten innerhalb eines Netzwerkes [Method for coordination of competing processes or for control of the transport of mobile units within a network]. Patent WO/2006/122528 (2006).
Networks for Transport, Supply, Information etc.: Universality, Robustness, and other Properties
56. Albert, L., Jeong, H. & Barabasi, L. A. Error and attack tolerance of complex networks. Nature 406, 378–382 (2000).
57. Polls, G. A. Stability is woven by complex webs. Nature 395, 744–745 (1998).
58. Milo, R. et al. Superfamilies of evolved and designed networks. Science 303, 1538–1542 (2004).
59. West, G. B., Brown, J. H. & Enquist, B. J. A general model for the origin of allometric scaling laws in biology. Science 276, 122–126 (1997).
60. Bettencourt, L. M. A., Lobo, J., Helbing, D., Kühnert, C. & West, G. B. Growth, innovation, scaling and the pace of life in cities. Proc. Natl. Acad. Sci. USA 104, 7301–7306 (2007).
61. Bebber, D. P., Hynes, J., Darrah, P. R., Boddy, L. & Fricker, M. D. Biological solutions to transport network design. Proc. Roy. Soc. B 274, 2307–2315 (2007).
62. Lämmer, S., Gehlsen, B. & Helbing. D. Scaling laws in the spatial structure of urban road networks. Physica A 363, 89–95 (2006).
63. Kühnert, C., Helbing, D. & West, G. B. Scaling laws in urban supply networks. Physica A 363, 96–103 (2006).
64. Helbing, D., Lämmer, S., Witt, U. & Brenner B. Network-induced oscillatory behavior in material flow networks and irregular business cycles. Phys. Rev. E 70, 056118 (2004).
Cellular Traffic
65. Howard, J. Mechanics of Motor Proteins and the Cytoskeleton (Sinauer Associates, Sunderland, 2001)
66. Morin-Ganet, M.-N., Rambourg, A., Deitz, S. B., Franzusoff, A. & Képès, F. Morphogenesis and dynamics of the yeast Golgi apparatus. Traffic 1, 56–68 (2000).
67. Komar, A. A., Lesnik, T. & Reiss, C. Synonymous codon substitutions affect ribosome traffic and protein folding during in vitro translation. FEBS Letters 462, 387–391 (1999).
68. Lesnik, T., Solomovici, J., Deana, A., Ehrlich, R. & Reiss, C. Ribosome traffic in E. coli and regulation of gene expression. J. theor. Biol. 202, 175–185 (2000).
69. Shea, T.B. & Beaty, W.J. Traffic jams: Dynamic models for neurofilament accumulation in motor neuron disease. Traffic 8, 445–447 (2007).
70. Parmeggiani, A., Franosch, T., Frey, E. Phase coexistence in driven one-dimensional transport. Phys. Rev. Lett. 90, 086601 (2003).
71. Nishinari, K., Okada, Y., Schadschneider, A. & Chowdhury, D. Intracellular transport of single-headed molecular motors KIF1A. Phys. Rev. Lett. 95, 118101 (2005).
Miscellaneous
72. Sellinger, A. et al. Continuous self-assembly of organic-inorganic nanocomposite coatings that mimic nacre. Nature 394, 256–260 (1998).
73. Adachi, M. & Lockwood, D. J. Self-Organized Nanoscale Materials (Springer, New York, 2006).
74. Nitta, T., Tanahashi, A., Hirano, M. & Hess, H. Simulating molecular shuttle movements: towards computer-aided design of nanoscale transport systems. Lab Chip 6, 881–885 (2006).
75. Bouchard, A. M., Warrender, C. E. & Osbourn, G. C. Harnessing microtubule dynamic instability for nanostructure assembly. Phys. Rev. E 74, 041902 (2006).
76. Alon, U. An Introduction to Systems Biology (Taylor & Francis, Boca Raton, 2007).
77. Schöll, E. & Schuster, H. G. Handbook of Chaos Control (Wiley-VCH, Weinheim, 2007).
78. Namatame, A. Adaptation and Evolution in Collective Systems (World Scientific, Singapore, 2006).
79. Wu, J. Distributed System Design (CRC Press, Boca Raton, 1999).
80. Dollimore, J., Kindberg, T. & Coulouris, G. Distributed Systems: Concepts and Design (Addison Wesley, Boston, 2005).